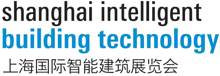
- Written by: Thomas Weise
Today, I attended the Shanghai Smart Home Technology (SSHT) [上海国际智能家居展览会] and Shanghai Intelligent Building Technology (SIBT) [上海国际智能建筑展览会] exhibitions in Shanghai. SIBT is an event for innovative intelligent building technologies and solutions related to the Internet of Things (IOT), cloud computing, and big data for building energy efficiency, energy management systems, and intelligent housing in general. SSHT, on the other hand, focuses more on home automation, technologies, technical integration, and cross-sector business collaboration in order to make our homes more convenient.
The exhibition was maybe slightly larger than the other fairs that I have visited this year and also had a higher visitor density. Also, almost all presenters at almost all booths there were permanently engaged in discussions with interested audience. It was clearly visible that there currently is a huge marked for smart homes and smart buildings with high consumer interest.
Many different companies presented diverse products. In my perception, the largest share of products were automation tools for end consumers such as automated switches maybe controlled via a mobile phone app, multi-functional sockets and switches with LCD displays, actuators such as automated curtain openers (maybe controlled via an app), intelligent locking and surveillance systems, and integrated home entertainment systems. There were providers of electronic building blocks for IOT applications, integrating, and controlling other systems for developing other applications and there were network infrastructure providers. Fewer, but present, were software vendors for building control and management software as well as home robotics.
I would say that the gravitational center of the exhibits was the convenience of the user. If we leave aside the new entertainment systems, I think most of the exhibitions were on better interfaces: nicer switches and apps with which you can turn on and off basically everything in your home. The fraction of truly intelligent systems, e.g., systems that would try to learn from and predict the user behavior or that would try to predict future events or changing environment conditions and adjust the home or building behavior to them, seemed to be smaller (but then again, I cannot really understand Chinese, so I may have overlooked some interesting exhibits).
Given the huge audience at the fairs and the market they represent, I would say that must be a huge future for intelligent homes and buildings. I saw some product frameworks which aim at the higher-level control over and the management of buildings and components. Given the already existing network infrastructure of the components, especially for large building compounds or office buildings (both of which we have many in China), intelligent technologies for water and energy saving, lighting or elevator control, automated cleaning robots, and maintenance scheduling will surely have a great market. And, of course, optimization, operations research, and machine learning could enable and improve on them.

- Written by: Thomas Weise
Optimization and Operations Research are about finding good solutions for computational hard problems. Sometimes we cannot guarantee to find the best solutions, because finding these (or the proof that they are best) may take too long. Then we look for good approximate solution to be found within reasonable time, i.e., our algorithm's performance is measured both in solution quality and required runtime (and runtime may be measured in different ways). Often we have algorithms that start with an initial (probably bad) solution and improve it over time. This is even often the case in situations where we can actually guarantee to solve the problem perfectly if we give enough time (and we may stop earlier once we run out of time, loosing the guarantee but at least having a solution). In Machine Learning and Datamining, the situation is quite similar, which is quite natural since many questions there could also be considered as special optimization tasks. In both fields, algorithms are often randomized, meaning that they may behave differently or return different results even if executed twice with the same input data.
From the above scenario, it becomes clear that it may not be easy to know which algorithm is actually the best for a given scenario. On one hand, we need to use some reasonably robust statistics. On the other hand, we may also need to clarify what "best" actually means, since we have at least two dimensions of performance (and reducing the algorithm performance to a single point measurement may lead to wrong conclusions). These are just two of the problems we face when evaluating new methods for optimization or Machine Learning. There are many more issues, such as how to compare multi-objective optimization methods (where we have more than one quality dimension) and how to compare parallel algorithms or programs running in a cloud or cluster? Or how can we compare algorithms on a problem which is noisy or in the face of uncertainties and the need for robustness?
There are many more opportunities for interesting and good research: How to visualize algorithm the results when comparing many algorithms on many problems? Can we model algorithm performance to guess how a method would perform on new problem? Can we have simple theoretical frameworks which gives us mathematically supported guidelines, limits, boundaries, or estimates for benchmarking? Or can we build automated approaches to answer high-level questions like: What features make a problem hard for a set of algorithms? Which parameters make an algorithm work the best? Are there qualitatively different classes of problems and algorithms for a certain problem type?
With our International Workshop on Benchmarking of Computational Intelligence Algorithms (BOCIA), we try to provide a platform to discuss such topics, a setup where researchers can exchange thoughts on how to compare and analyze the performance of Computational Intelligence (CI) algorithms. We generously consider all optimization, Operations Research, Machine Learning, Datamining, and Evolutionary Computation all as sub-fields of CI. This workshop will take place at the Tenth International Conference on Advanced Computational Intelligence (ICACI 2018) on March 29-31, 2018 in Xiamen, China.
If you are a researcher working on any related topic, we would be very happy if you would consider submitting a paper until December 1, 2017, via the submission page. Here you can download the Call for Papers (CfP) in PDF format.
Read more: Workshop on Benchmarking of Computational Intelligence Algorithms approved for ICACI 2018

- Written by: Thomas Weise
at the Tenth International Conference on Advanced Computational Intelligence (ICACI 2018)
March 29-31, 2018 in Xiamen [厦门], Fujian [福建省], China
http://iao.hfuu.edu.cn/bocia18
BOCIA, the International Workshop on Benchmarking of Computational Intelligence Algorithms, a part of the Tenth International Conference on Advanced Computational Intelligence (ICACI 2018), was cordially inviting the submission of original and unpublished research papers. It took place on March 31st, 2018.
Computational Intelligence (CI) is a huge and expanding field which is rapidly gaining importance, attracting more and more interests from both academia and industry. It includes a wide and ever-growing variety of optimization and machine learning algorithms, which, in turn, are applied to an even wider and faster growing range of different problem domains. For all of these domains and application scenarios, we want to pick the best algorithms. Actually, we want to do more, we want to improve upon the best algorithm. This requires a deep understanding of the problem at hand, the performance of the algorithms we have for that problem, the features that make instances of the problem hard for these algorithms, and the parameter settings for which the algorithms perform the best. Such knowledge can only be obtained empirically, by collecting data from experiments, by analyzing this data statistically, and by mining new information from it. Benchmarking is the engine driving research in the fields of optimization and machine learning for decades, while its potential has not been fully explored. Benchmarking the algorithms of Computational Intelligence is an application of Computational Intelligence itself! This workshop wants to bring together experts on benchmarking of optimization and machine learning algorithms. It provides a common forum for them to exchange findings and to explore new paradigms for performance comparison.
Here you can download the BOCIA Workshop Call for Papers (CfP) in PDF format and here as plain text file.
All accepted papers in this workshop will be included in the Proceedings of the IEEE ICACI 2018 published by IEEE Press and indexed by EI. Authors of selected papers will be invited to submit extended versions of these papers to the Special Issue on Benchmarking of Computational Intelligence Algorithms in the Applied Soft Computing journal by Elsevier B.V., indexed by EI and SCIE. Here you can download the Special Issue Call for Papers (CfP) in PDF format and here as plain text file.
Read more: International Workshop on Benchmarking of Computational Intelligence Algorithms (BOCIA)
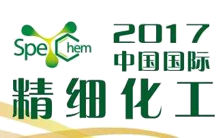
- Written by: Thomas Weise
Today, I attended the China International Chemical Industry Fair (ICIF China) [中国国际化工展览会] and SpeChem [中国国际精细化工及定制化学品展览会] exhibitions in Shanghai, i.e., trade shows for the chemistry industry. ICIF is a general fair for all types of chemicals and chemistry-equipment, ranging from petroleum to fertilizer and from pumps and valves to packaging and logistics. SpeChem is focused on the special chemicals used in, e.g., the food and beverage, pharmaceutical, agricultural, electronic, water treatment, oilfield, paper, and textile industries. After already attending several logistics-related events this year, such as Intermodal and LogiMAT, I wanted to attend this fair in order to broaden my focus and to see whether IT and operations research have become central products in the chemistry industry.
The exhibition was quite large and a wide variety of products were presented. Manufacturers of organic and inorganic chemicals promoted their products, but also machinery, be it for cleaning water, automatic packaging, or producing chemicals were displayed. Hoses, valves, vessels, canisters and other utilities could be found. As a side node, I was positively surprised to find quite a lot of Anhui-based companies at the exhibition. Still, most interesting for me were producers of automation and control equipment as well as logistics providers. I even had the chance to discuss with a few of them.
The manufacturers of automation equipment for chemistry production currently seem to mainly focus on traditional features, such as fixed control procedures and networking of components, which, well, is their basic business. However, some of them seriously consider adding more intelligence to their products and production processes, be it by enhancing existing products with networked sensors, making their own production "smart," or by contemplating adding intelligent control and planning capabilities to their products. The logistics providers in the chemistry sector will need to rely on manual negotiations in the foreseeable future due to the volatility of the market and ever-changing regulations. Yet, providers for chemical freight tracking software may begin to utilize Big Data approaches to mine and provide useful information for their clients. In summary, at least from the few discussions I had, I think the chemistry industry is not yet widely utilizing the available capabilities of IT and computational intelligence, although several of my interlocutors think that this will take place in the future, will be a necessary development, and/or are seriously planning to move into this direction.
I believe that the chemistry industry could largely profit from a movement similar to Industry 4.0 (may I dare to call it Chemistry 4.0?). Often, they deal with automated processes run by huge machinery. Questions such as the automated configuration of their process parameters and the best scheduling of production tasks and maintenance works arise quite naturally. Also, chemical manufacturers often do not produce based on customer orders but perform stockpiling, which asks for intelligent predictions of future orders. All of the above could be single optimization problems or even combined into a complex integrated optimization task, maybe even involving optimization along the whole supply chain. Of course, to get there means to first invest money into the development of optimization tools, without clearly knowing how much resources or money can be saved or how much the productivity can be increased beforehand. This may be an understandable and large hurdle, nevertheless, I believe that there is a huge potential in this field.
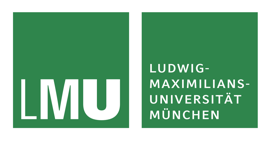
- Written by: Thomas Weise
On August 3, 2017, I presented my research talk Automating Scientific Research in Optimization at the Database Systems and Data Mining Group of Prof. Thomas Seidl at the Institute of Informatics an the Computational Statistics Group of Prof. Dr. Bernd Bischl who is at the Institute of Statistics, both of the Faculty of Mathematics, Informatics, and Statistics of the Ludwig-Maximilians-Universität Munich (LMU) in Munich (München), Germany. The talk was organized by Dr. Markus Mauder.
The Database Systems and Data Mining Group conducts research on data science, data mining, machine learning, artificial intelligence and database technologies. They conduct both fundamental and applied research and aim to supporting partners from many domains such engineering, business, humanities and life sciences in analyzing huge and complex data. Their research topics include explorative data analytics (clustering, outlier detection and interactive data mining), new computation models for data mining (cloud architectures, MapReduce, deep learning, GPU computing, embedded analytics), data mining for complex objects (spatio-temporal data, graphs and networks, multimedia, community detection, trajectory analysis, network queries, pattern mining, dealing with uncertain data, as well as games and sports analytics), process mining (temporal aspects in process models, event identification, process model identification, stream process mining), and similarity search. Since my presentation is mainly on applying data mining and machine learning to extract information from experiments with optimization methods, this provided good grounds for nice discussions after the presentation. They collaborate with partners like Siemens and BMW.
The Computational Statistics Group conducts research in predictive modelling (e.g., non-linear classification and regression), ensemble techniques, statistical and machine learning, statistical computing and computational statistics, model- and variable selection, expensive black-box optimization, bayesian optimization, and statistical software development. They organize the Munich City R courses, R being the one of the most important statistics software packages out there right now and is also used inside of our optimizationBenchmark software. They have contributed a huge set of awesome software packages, including, for instance, a machine learning toolbox for R and an R package for the COCO framework (one of the works related to our talk).
Prof. Bischl is furthermore one of the founders of COSEAL, the COnfiguration and SElection of ALgorithms group, an international group of researchers with focus on algorithm selection and algorithm configuration. These two topics are the exact complement of what I presented in my talk: While my system analyzes how algorithms behave and tries to find reasons (which a researcher can use to improve the algorithm), the work of COSEAL is trying to find ways to automatically find/choose the best behaving algorithms (or algorithm configuration). The group is very active and organizes workshops, competitions, and provides software and benchmark datasets on their fields. (In September 2017, I became a member of COSEAL.)
Giving a presentation at LMU with attendants from both groups was very exciting for me. I came here with the goal to broaden my horizon and to learn where these experts see merits and errors in my presented approach. The after the presentation discussion was indeed very interesting and fruitful.
- Research Talk "Automating Scientific Research in Optimization" by Prof. Thomas Weise at the Johannes Gutenberg University Mainz in Mainz, Germany
- Research Talk "Automating Scientific Research in Optimization" by Prof. Thomas Weise at the University of Cologne in Köln (Cologne), Germany
- Research Talk "Automating Scientific Research in Optimization" by Prof. Thomas Weise at the University of Leipzig in Leipzig, Germany
- Research Talk "Automating Scientific Research in Optimization" by Prof. Thomas Weise at the University of Applied Sciences Zittau/Görlitz in Görlitz, Germany