- Written by: Thomas Weise
On January 20, 2020, our secretary Mrs. Zhou Bing [周冰] and Prof. Dr. Thomas Weise [汤卫思] attended the New Year's Party for the Friends of the Hefei Foreign Affairs Office [合肥市人民政府外事办公室]. The meeting was hosted by Mr. Qiliang Chen [陈启亮], director of the Hong Kong and Macao Affairs Office [合肥市人民政府港澳事务办公室]. Most of the attendees were overseas Chinese who return home to celebrate the Spring festival together with their families. Even though they now live in countries such as the USA, Spain, Hungary, Belarus, Russia, or Argentina, the ties to their hometown remain. This was a very happy evening with a nice dinner and a chance to meet new friends.
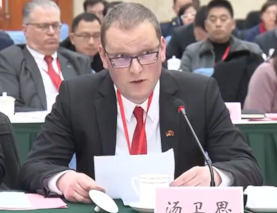
- Written by: Thomas Weise
One January 19, 2020, I attended the 2020 Tea Party for High-Level Talents and Symposium for Newly Elected Academicians [2020年高层次人才迎春茶话会暨新当选院士座谈会] of the province Anhui [安徽省] held in the East Hall [东楼礼堂] of the Daoxianglou Hotel [稻香楼宾馆]. In this meeting, the seven(!) new academicians of our province as well as high-level talents and teams were introduced. The importance of science, technology, and the cultivation of top-level talents in all fields was emphasized. Mr. Jinbin Li [李锦斌], the party secretary of our province, as well as Mr. Guoying Li [李国英], the province governor, both took part in this meeting, which further stresses the deep interest of the province leadership in pushing technological and scientific progress.
- Written by: Thomas Weise
One January 10, 2020, foreign experts of Hefei University [合肥学院] met with our new president, Prof. Dr. Chunmei WU [吴春梅]. Our group of foreign experts taking part in the meeting included Prof. Falk Höhn of the Chinese-German Institute of Applied Sciences, Hefei University [合肥学院中德应用科学学院] and Professor of Industrial Design, Prof. Minyong Da [闵泳大], Professor of Korean Language and Literature, Mr. Olaf Rack, Lecturer of Industrial Design, Mrs. Jutta Lahmann and Mr. Adrian Lempert, German language lecturers, and Prof. Thomas Weise [汤卫思], Director of the Institute of Applied Optimization [应用优化研究所]. The keen interest of Prof. WU in our work and in supporting our work was very encouraging and emphasized by the fact that meeting and discussing with us was one of the first actions she took after just recently accepting the position as president of Hefei University. The meeting was very pleasant and featured an open and very constructive environment in which our future plans for further improving the internationalization and teaching at our university were discussed. It should be noted that every single expert expressed great satisfaction with the working and living environment provided by our university as well as gratitude for support that we received during all the years working here.
Read more: Foreign Experts meet with President Prof. Dr. Chunmei WU

- Written by: Thomas Weise
On January 6th, 2020, Mr. Kaiqiang HUANG [黄凯强] of TU Dublin, Dublin, Ireland gave his research talk Human Action Recognition using Zero Shot Learning [零次学习运用于人体动作识别] at 14:30 in our meeting room 920 in our office building 53 [合肥学院53 栋920]. Mr. Huang received his Bachelor from our university [合肥学院] and TU Dublin in 2016 before doing his Master's at TU Dublin, where he now has become a fully-funded PhD student. His talk, introducing a method to combine semantic side information with learned human actions was highly exciting for the audience. In the over 15 minutes of discussion that followed, we delved into the depths of this interesting topic, which is directly relevant to the work of several of our team mates. I think our school can be proud of Mr. Huang, who has grown very much and has become an excellent researcher in such a short time.
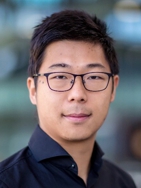
- Written by: Thomas Weise
On January 6th, 2020, Dr. Bin CHEN [陈斌] of Eindhoven University of Technology, Eindhoven, The Netherlands, and Hefei University of Technology [合肥工业大学] gave hold research talk Multidimensional Modulation Formats for High Speed Optical Communications [面向高速光通信的多维度调制技术] at 15:30 in our meeting room 920 in our office building 53 [合肥学院53 栋920]. Today, the internet is a vital part of the infrastructure and subject to an ever-rising demand for bandwidth, much of which is routed through optical-fiber based connections. In his highly interesting talk, Dr. Chen discussed the limits of this technology and how we can push them further. If our national and international network connectivity can keep growing and improving in the future, one of the reasons may well be the work of Dr. Chen, which won two best paper awards. Thanks for this great talk!
- Open Optimization Competition 2020
- Good Benchmarking Practices for Evolutionary Computation (BENCHMARK@GECCO) Workshop
- Good Benchmarking Practices for Evolutionary Computation (BENCHMARK@PPSN) Workshop
- Second Institute Workshop on Applied Optimization: Seminar on Operations Research and Optimization